Cardiac-Kidney-Metabolic Health and Unsupervised Learning
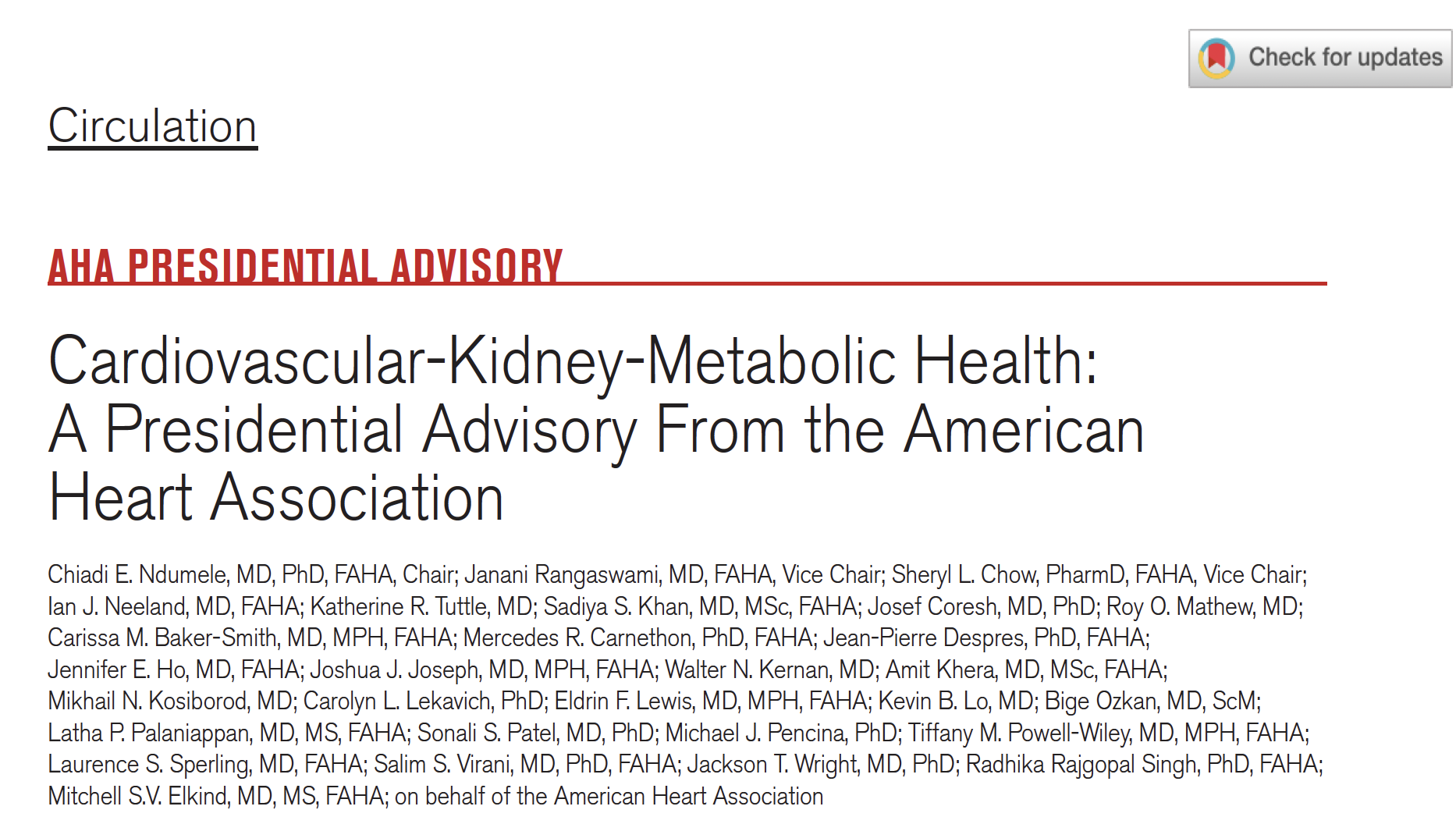
Almost eight years ago, my close collaborator Sanjiv Shah and I published a manuscript applying unsupervised machine learning techniques to data from patients with heart failure with preserved ejection fraction (HFpEF). The rationale for our work was that HFpEF is so loosely defined that it represents an ineffective categorization for clinical decision-making — as it groups together individuals with widely different prognoses. Our data-driven approach resulted in more homogenous clusters of individuals, differing by electrical, biochemical, and structural features, and clinical outcomes. Moreover, there was an additional suggestion that the biology underlying such classes might differ.
This work and related manuscripts by others brought to the forefront the question of the value of such groupings. The most suspect claim (which I admit to making) is that the underlying disease pathogenesis for such complex conditions is likely to be different and can be neatly binned. Having spent over 15 years of my life studying genetics scientifically, a decade of seeing patients with inherited cardiac disease, and many years running a developmental biology laboratory, I am increasingly skeptical that adult-onset diseases can be neatly grouped by underlying pathophysiology. There are simply far too many complex environmental and genetic factors interacting over decades and decades to believe there are a finite number of dominant attractor states. My developmental biologist colleagues at UCSF, who were superb scientists, used to discourage characterizing model organisms beyond a few days of development, as the system becomes so complex that all bets are off on trying to build a reductionist case for what factors drive the resulting phenotype. If so, what hope do we have of making mechanistic claims for 60-year-old humans?
For me, these somewhat philosophical thoughts (which conjure up dreadful words like nosology) awaken now and then but are quickly swatted away by the all-consuming challenges of starting a health-tech company. What has led me to consider them again is the recent articles on Cardiac-Kidney-Metabolic (CKM) syndrome, a newly-defined syndrome, featuring staging reminiscent of heart failure and valvular disease, and coupled with new equations for prediction of adverse cardiovascular events.
As a company we are delighted to see this heightened awareness, as much of our software platform has been focused on the CKM space, with our software elevating mid-level practitioners to achieve specialist-level decision-making for cardiac (heart failure, hypertension, hypercholesterolemia, atrial fibrillation, …), metabolic (obesity, type 2 diabetes) and kidney (CKD, hyperkalemia, hyperphosphatemia, anemia of CKD, secondary hyperparathyroidism …) diseases. We are also heartened by the emphasis on SDOH factors, which our care navigators systematically collect data on and address in terms of overcoming barriers to reaching therapeutic targets.
But it does seem that many of the same topics as with HFpEF are being considered around underlying biology and pathophysiological mechanisms, seeking some sort of dominant drivers of disease and parsimony. As I mentioned, I am skeptical there is much to be found there.
However, where I see the need for a syndrome is to highlight that high-risk patients with multiple, complex, comorbid conditions such as CKM patients do terribly in the current healthcare system, repeatedly falling through the cracks. They are often too complex for primary care physicians — given the rapid introduction of new medications, the number of concurrent diseases, and the concerns for major adverse events. But specialists do not usually have the bandwidth to implement the high-touch iterative care needed to get patients on the right medications at the right doses, wrestle with inevitable side effects, and help patients with the many SDOH obstacles that may present along the way. And there is a nihilism around prevention, because risk arises from multiple different factors, but no single attribute is so overwhelmingly bad to drive action.
Unfortunately, current fee-for-service reimbursement models do not incentivize solving these problems. As a result, we have put our company’s focus on value-based-care models that recognize the need to get these patients to therapeutic goals, including systematically identifying and overcoming whatever barriers there may be. Perhaps the greatest value of the CKM syndrome is that its definition exposes a healthcare system that is bound to fail these patients unless new innovative approaches are taken.
Rahul D.