My difficulties with GPS (a thinly-veiled parable about AI and healthcare)
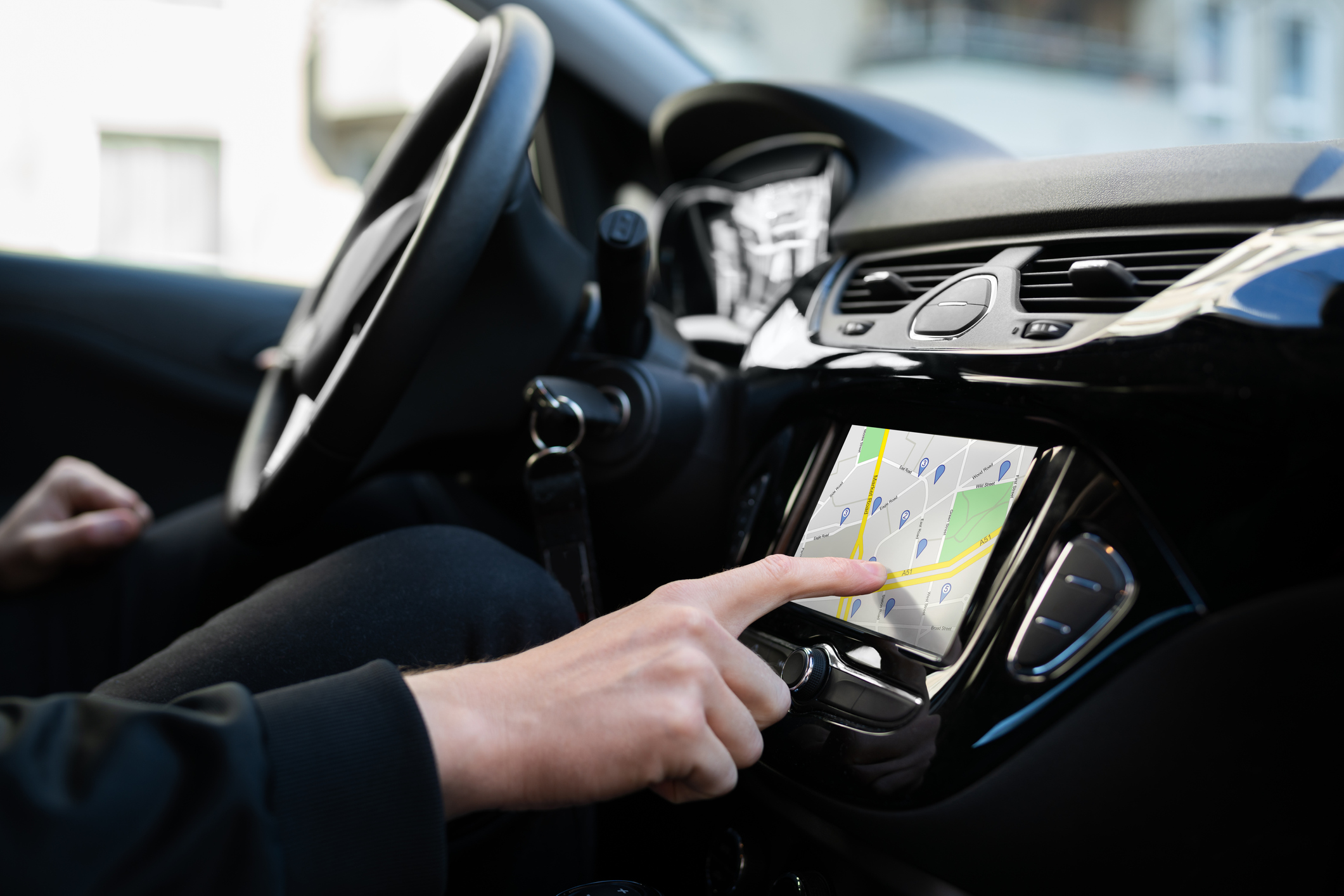
I have a middling sense of direction. Actually, I have a very poor sense of direction, but it has been covered up well with my constant use of GPS. But the result is that I’ve now become so dependent on GPS, I use it even on routes I’ve traveled hundreds of times before.
Other than a minor bit of embarrassment, this solution seems acceptable. But as has been described, I have no doubt the use of this “co-pilot” has eroded my natural abilities to navigate independently. There are many occasions where GPS fails us (my kids will tell you it’s at the I-90:I-93 junction) and in those settings I reliably unravel. And as you might expect, I am useless at explaining to anyone else how to get from point A to B.
You might wonder what the point of this story is. Well, “co-pilots” in AI are all the rage these days. But as was seen with computer-assisted mammography interpretation, which has been around for decades, there was no obvious improvement in detection with their use and some concern that readers actually performed worse when using them. One of the reasons for this is that the AI software makes no effort to improve the human’s ability to carry out the same task and may, in fact, interfere with their usual process. It’s every man and machine for themselves. In fact, as we have moved to more complex neural network architectures, which likely deviate substantially from how humans learn, there is less hope we will be able to understand how the machine arrived at its interpretation. And if that is the case, what hope do we have to explain to patients what our reasoning is, why we are recommending some course in the face of uncertainty, and how they can work through the same reasoning process to feel confident about the risks they are taking on. Routinely, I (and our navigators) get questions from patients such as “What about me makes this the best decision for me?”. The expectation is that the answer will boil down to one or two or, at most, a handful of interpretable characteristics they recognize about themselves.
I’ve faced this tension with much of my early research in the field on electrocardiogram interpretation. Although the renaissance of AI in the past decade has emphasized not attempting to emulate how humans perform a similar task, I suspect this approach will face an uphill battle if AI products expect to shape decisions that must be conveyed to understandably skeptical patients – which, for our field (though perhaps not for radiologists), is nearly every single one. There may be tolerance for a complex model here and there (e.g., genomic breast cancer risk markers, which are nonetheless still interpretable) – but even in that case it’s a small part of a risk equation, with the other features all being explainable to patients.
Leo Breiman wrote perceptively wrote about this conundrum over 20 years ago. I see obvious relevance to the recent interest in LLMs. As I’ve described in other forums, I expect (putting my nickel down) much of this LLM focus to have only a modest upside in medicine, primarily in applications peripheral to clinical decision-making, with benefits plateauing quickly.
If I were to build a GPS system, I would have it teach the user how to improve their own sense of direction with every use. And it would give the user tools to explain their reasoning to others. When it comes to healthcare, there are obvious parallels. The ideal AI-driven software would enable everyone (physician, navigator, patient, family caregivers) to share in a common transparent cognitive model of health, disease, and decision-making under uncertainty.
Rahul D.